In Case You Missed It: Ample Insight’s CEO Garry Ma, delivered a webinar in partnership with Communitech, focusing on the critical role of data quality in building trusted AI. With his extensive background as an early data scientist at Facebook (Meta), co-founder of an AI recruiting solution, and current AI business consultant, Garry is uniquely qualified to discuss this topic. Let’s dive into the details:
Why Data Quality Matters
As AI continues to grow exponentially, its foundation -data- becomes increasingly important. AI’s outputs are only as good as its inputs, making data quality a vital consideration for organizations. The figures below illustrate the significant potential for improvement in many organizations.
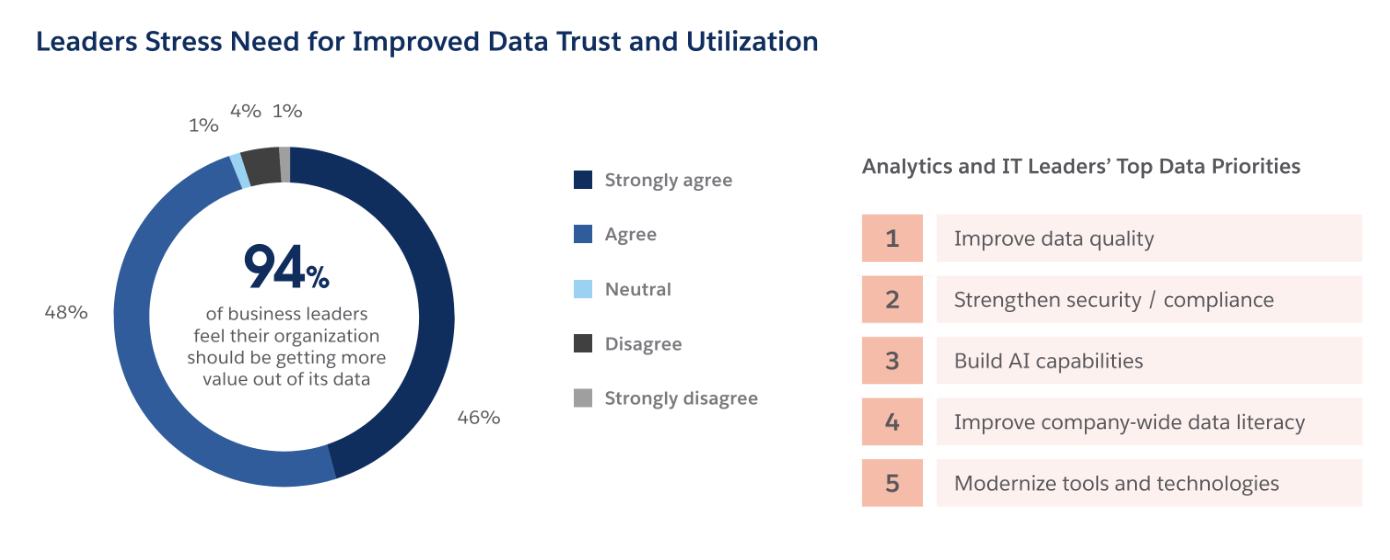
Dimensions of Data Quality
The terms Validity, Consistency, Completeness, Timeliness, and Uniqueness all define data quality. Let’s dive deeper into these dimensions to develop a comprehensive idea of data quality.
- Validity: Data must conform to the syntax (format, type, range) of its definition. For example, date fields should only contain valid dates, not text or numbers.
- Consistency: Data should align across datasets without contradictions. For example, addresses between different database tables should have the same format.
- Completeness: Data should include all necessary parts, with no missing values. For example, required contact information for a customer database should not be missing fields.
- Timeliness: Data must be available when needed and reflect the current state. For example, sales data in a data warehouse should not be days behind.
- Uniqueness: Data should not contain duplicate entries. For example, each customer should have a unique identifier to prevent duplicates in the system.
Strategies for Data Quality
Ensuring data quality is a collective responsibility that requires alignment among all data stakeholders. Here are five strategies to enhance your data quality:
- Create Data Governance Standards: Establish clear guidelines and policies for data management.
- Profile and Clean Data: Regularly examine and cleanse data to maintain its quality.
- Implement Data Validation and Monitoring: Continuously validate and monitor data to ensure data accuracy and integrity.
- Train Staff on Latest Data Workflows & Policies: Educate stakeholders on principles, policies and their role in maintaining data quality.
- Make Feedback Easy & Continuously Improve: Foster a culture of continuous improvement and regularly gather input and updating processes to enhance data quality.
Landscape of Data Tools
Using the right tools is essential for improving data health. When assessing your data health, consider the following aspects and their associated features:
Data Quality
- Data profiling
- Data issue detection
- Data issue monitoring
Data Observability
- Data discovery & cataloguing
- Data lineage mapping
- Data pipeline monitoring
Data Cataloguing
- Centralized inventory of data assets
- Metadata management
- Data discovery
Data Fabric
- Unified data management & access
- Data observability and cataloguing

Building Trusted AI
“Building good AI is like preparing a nutritious meal.”
Several principles have been defined by various governments and organization for building responsible AI, however, the core principles are:
- Transparent, Explainable, and Accountable
- Safe and Sensible for Human, Society and Environment
- Fair and Human-centred Values
Industry concerns have emerged about the ethical operationalization of generative AI. While 83% of IT leaders believe companies must collaborate to ensure the ethical use of generative AI, only 30% feel ethical use guidelines are a requirement for successful implementation.
Conclusion
This webinar demonstrated that data quality is at the cornerstone of trusted AI. It’s important to remember that investing in your data quality is a continuous process, and it is never too late to start.
Be mindful and create Good AI.
Additional Resources
Watch the Good AI Webinar recording here.
For additional information about data quality and building trusted AI, check out these supplementary materials:
- State of Data and Analytics by Salesforce (2023)
- The Executive’s AI Playbook by McKinsey
- Building a High Performance Data and AI Organization by MIT Technology Review
- Principles for Ethical Use of AI by Government of Ontario [Beta]
- Ethics of Artificial Intelligence by UNESCO
References
- Taylor, P. (2023). Amount of Data Created, Consumed, and Stored 2010-2020, with Forecasts To 2025. Statista. https://www.statista.com/statistics/871513/worldwide-data-created/
- The Executive’s AI Playbook. QuantumBlack AI by McKinsey. https://www.mckinsey.com/capabilities/quantumblack/our-insights/the-executives-ai-playbook?page=industries/
- Batchelder, W. (2023). State of Data and Analytics. Salesforce. https://www.salesforce.com/content/dam/web/en_us/www/documents/research/state-of-data-analytics.pdf
- Ethics of Artificial Intelligence. UNESCO. https://www.unesco.org/en/artificial-intelligence/recommendation-ethics
- Principles for Ethical Use of AI [Beta]. Government of Ontario. https://www.ontario.ca/page/principles-ethical-use-ai-beta
- Australia’s AI Ethics Principles. Government of Australia. https://www.industry.gov.au/publications/australias-artificial-intelligence-ethics-framework/australias-ai-ethics-principles